A warmer climate can affect the kind of cloud cover we get, and the cloud cover can in turn affect the climate.
Low, heavy clouds reflect a lot of sunlight and can have a cooling effect on the climate, while thin clouds at high altitudes can capture heat radiation from the earth, leading to warming.
There is much uncertainty related to what the formation of clouds will be like in a warmer climate, and how much of the sky will be covered by them.
Changes in cloud cover can affect global warming, which in turn affects important resources such as agriculture and solar energy.
Machine learning makes it easier to predict cloud cover
Researchers from OsloMet, SimulaMet and the University of Oslo have now developed a data set that can use machine learning to see connections between cloud cover over Europe and air temperature, air pressure and air humidity.
This can provide better forecasts of what may happen to the climate and cloud cover in the future.
“As far as we know, this is the first data set that can use machine learning to predict cloud cover,” says professor at OsloMet and machine learning expert, Hugo Hammer.
The dataset consists of satellite observations of cloud cover, in addition to observations of air temperature, air pressure and air humidity.
The researchers propose a new technique that can link satellite observations, air temperature, air pressure and humidity to specific geographical areas.
One challenge is that the satellites observe different parts of the earth's surface at different angles. This causes the resolution to vary, and this technique compensates for this.
This particularly applies to observations of the cloud cover towards the poles, which receive lower resolution, while there is the best resolution and most observations around the equator.
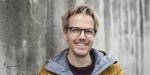
"We can say something about how different values of temperature, pressure and humidity appear at the same time as certain types of cloud formation," says Professor Hugo Hammer. Photo: Sonja Balci, OsloMet
First to predict cloud formations in the future
Hammer sees many exciting issues related to machine learning and statistical methods in climate research and weather forecasting.
So far, machine learning, especially deep learning, has not been used that much in climate research compared to weather forecasting, for example.
The work to create Europe's first data set to predict cloud cover stems from a master's project, where Hugo Hammer, Michael Riegler and Trude Storelvmo supervised master's student Hanna Svennevik at the University of Oslo.
“If we use a good climate model as a starting point, we can use our dataset to predict the degree of cloud cover in the future.”
“For example, if a climate model tells us estimates of values for temperature, air pressure and humidity in 2060, we can put these values into a model trained on our data set to say something about the degree of cloud cover in 2060.”
A similar example could be that if the climate model simulates up to the year 2100, then one can take modelling of temperature, air pressure and humidity for the year 2100, put it into the trained model, and make a prediction of cloud cover in the year 2100.
“We cannot say how pressure and temperature will necessarily affect clouds, but we can say something about the extent to which different values of them appear at the same time as certain types of cloud formation.”
Uncertainty in machine learning
“We can then learn that there are co-variations between temperature, air pressure and humidity on the one hand and cloud cover on the other.”
Co-variation then means that certain values of temperature, air pressure and humidity occur at the same time as certain types of cloud cover.
“However, our data set is based on historical data. If the climate changes in the future, the relationship between temperature, air pressure, humidity and cloud cover may also change. This could make a machine learning model trained on the historical data less precise.
A weakness of machine learning is that when you put in data that is slightly different from what was used when the model was trained on this data, it becomes more uncertain. It then becomes more difficult for the model to predict what will happen.
For example, if you train the model with temperatures of 16 to 30 degrees, and then suddenly put 40 degrees into the model, it will still predict the cloud cover. However, it will be less reliable because we had no data to train how the cloud cover behaves at 40 degrees. We don't know for sure because we didn't have those observations.
The predictions will therefore be somewhat uncertain anyway. At the same time, the researchers’ data set can contribute to better predictions, as it provides more knowledge about the effects of temperature, humidity, and air pressure.
Temperature seems to be most important
“So far, what seems to be most important for predicting cloud cover?”
“Temperature appears to be most important. Humidity is in second place, and air pressure is third.”
Temperature is important for several reasons. Firstly, clouds form when moist air cools. Secondly, the temperature is decisive in terms of how much water vapour the air can contain. Thirdly, the temperature at different altitudes in the atmosphere affects cloud formation.
Clouds also have a high degree of humidity, and cloud cover is closely related to air pressure, as clouds often form in areas of low pressure.
However, the relationship between these is complex, and is affected by a number of factors.
Best to start from a limited area
“Why have you limited yourself to Europe?”
“It is very common to limit yourself to a defined geographical area. Weather and climate can be very different in different parts of the world, and many issues arise if you try to do it on a global scale.
Therefore, it is common to focus only on one area,” says Hugo Hammer, who recommends other researchers to use the data set.
References
Hanna Svennevik, Steven A Hicks, Michael A. Riegler, Trude Storelvmo and Hugo L. Hammer: A dataset for predicting cloud cover over Europe. Scientific Data 11, Article number 245 2024).